No Language Left Behind
Four billion people around the world speak languages not served by Siri, Alexa, or chatbots—to the detriment of global public health, human rights, and national security. Here’s how our experts are leveraging artificial intelligence to achieve digital equity for people the world over.
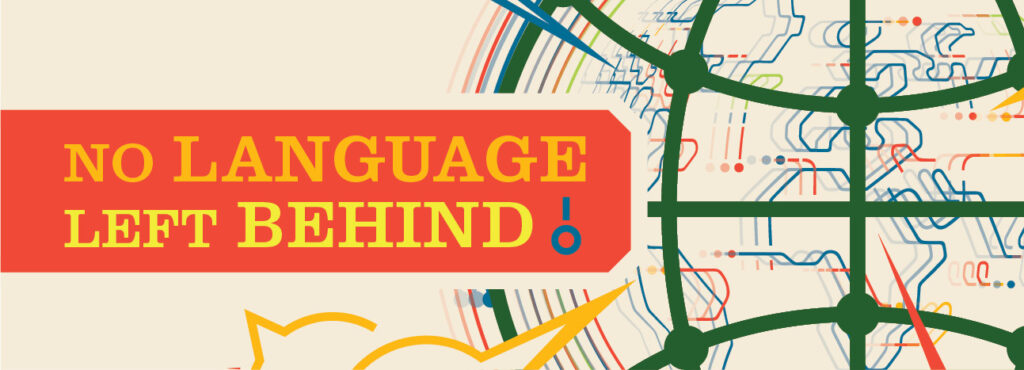
In 2010, an earthquake devastated Haiti. Relief workers poured in from around the world. Makeshift cell towers were hastily raised to reestablish phone service. Desperate calls for help came from every direction, yet many went unheeded.
“Not from a lack of relief workers, but because no one spoke Haitian Creole,” recalls Matthew Wiesner, a research scientist at the Center for Language and Speech Processing at Johns Hopkins Whiting School of Engineering.
Wiesner first learned of the Haitian example in 2016 while contributing to a U.S. Department of Defense initiative known as Low Resource Languages for Emergent Incidents (LoReLEI), whose aim was to help translate any language in as little as 24 hours after first encountering it.
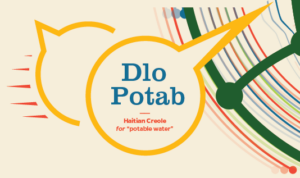
The urgency of the Haitian example highlights the complex challenges experts at CLSP have grappled with for decades—bringing the power of natural language processing, like that behind Alexa, Siri, and ChatGPT, to the world’s lesser-spoken languages. Depending on whom you ask, there are between 6,000 and 7,000 languages spoken on planet Earth today. As remarkable as that number is, experts estimate that half the world’s people speak just 100 or so languages. That leaves a staggering four billion people speaking any one of the thousands in that long list of rare languages.
“That is a vast underserved population,” says Sanjeev Khudanpur, an associate professor of electrical and computer engineering who leads the Center for Language and Speech Processing. “Many languages beyond the top 20 or 30 most-spoken languages don’t work with Siri or Alexa. No one is developing chatbots in these languages.”
A fair number of the 3,000 least-spoken languages in the world are so rare that they are in danger of becoming lost forever as the relative handful of speakers dwindles and no one steps in to keep the languages alive. Many Native American languages are endangered. Younger generations aren’t learning them. These languages don’t work on smartphones or proliferate on the internet.
Other languages present yet different challenges. Several thousand languages are rarely written and many lack a standardized writing system. Others lack digital alphabets to preserve them on computers where they can become valuable data sources. Such is the case in South Asia. The Devanagari alphabet exists on most operating systems, but is not as widespread as the Latin alphabet. And even then, less than half of South Asians speak languages written in Devanagari. Collectively, the population speaking languages lacking suitable digital alphabets could number a few billion people, Khudanpur says.
“Meanwhile voice recognition, translation, and natural language processing using AI are changing the world, but only for a select few languages,” Khudanpur says. “If the language you speak is among the thousands of outliers, AI is not for you.”
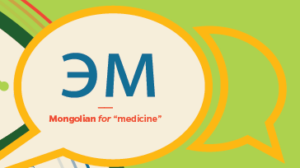
It is a matter of digital equity, Khudanpur stresses. Today’s ubiquitous voice recognition applications, like Alexa and Siri, and artificially intelligent chatbots, like ChatGPT, are concentrated on a relative handful of the most-spoken languages—English especially. As AI becomes more powerful, the world’s most-common languages will benefit. The rest will fall behind. Too many will die.
“If a Quechuan kid in Peru can’t surf the web in Quechua, they’ll use Spanish,” says David Yarowsky, a professor of computer science and a computational linguist on the CLSP faculty. “This is a rapidly accelerating train.”
The Center for Language and Speech Processing is one of the foremost academic research centers in the world studying computerized language processing, automatic speech transcription, and machine translation. The center conducts research in many relevant areas including acoustic processing, automatic speech recognition, cognitive modeling, computational linguistics, information extraction, machine translation, and text analysis, among others.
Khudanpur’s handiwork is behind the voice recognition algorithm in Amazon’s Echo—better known as Alexa. He imagines a world where computers and smartphones can instantaneously hear or read any language in the world, understand what’s been said, and then translate those words into any other language on Earth instantaneously—an Amharic Alexa, a Chechen ChatGPT. But that dream is far, far off, he says.
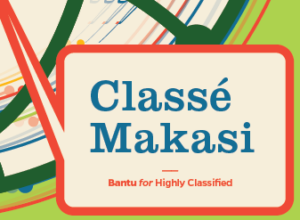
Khudanpur and his colleagues at CLSP are applying their myriad skills to extend AI’s reach to those underserved billions. The cause is one of cultural preservation, but increasingly, Khudanpur says, also a matter of national security, of global public health, and of human rights.
The National Science Foundation has taken particular interest in studying these so-called “underresourced languages” to preserve their cultural histories. Despite the concerted efforts of CLSP and others in academia and in industry to support them, many languages will fall silent, but the work could at the very least preserve them for posterity, the way archeologists, classicists, and anthropologists learn Latin, ancient Greek, hieroglyphs, and Old English to extract meaning from important cultures of the past.
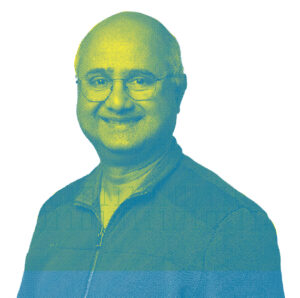
“Many languages beyond the top 20 or 30 most-spoken languages don’t work with Siri or Alexa. No one is developing chatbots in these languages.”
— Sanjeev Khudanpur
“Loss of a language is loss of knowledge,” Khudanpur says. “The Eskimo have some 50 words for snow. Perhaps one describes ice too thin to walk on.”
The U.S. Department of Defense considers the study of rare and dying languages as a matter of national security. LoReLEI is but one example. Recall the urgent pleas for speakers of Urdu, Pashto, and tribal languages of Afghanistan and Pakistan in the early 2000’s Afghanistan War. Understanding a rare language might distinguish a recipe for a babka from a recipe for a bomb or unlock a trove of information in a captured laptop. In a public health setting, knowing a rare language might help beat back the next pandemic or speed relief to victims of natural disasters like those victims of the Haitian earthquake whose calls for help went unmet.
“Johns Hopkins is one of the major forces in trying to provide the language technologies that will help make the thousands of languages remain viable,” Yarowsky explains.
HOLY WORDS
Like many at CLSP, Yarowsky uses machine learning to study the structure and rules of language. Increasingly, the challenge comes down to data, or the lack of it—a deficit experts are referencing when they describe a language as “underresourced.” There aren’t vast stores of written text or hours of recorded speech to work from. Books and other materials that are a rich resource for the more widespread tongues are rarely translated into the lesser-spoken languages.
“There’s a very long tail of languages that get left behind. And unfortunately, resources like GPT-3 and technologies like ChatGPT, Alexa, and Siri, even the internet itself, are making the digital divide worse,” Yarowsky says.
GPT-3, the large language model behind ChatGPT’s “intelligence,” contains some 250 billion words of written text—almost all in English. That’s more than 900,000 Bibles
worth of written content, which just happens to be Yarowsky’s go-to data source. The Bible has been translated into more than 1,500 languages by missionaries evangelizing in remote corners of the globe.
Yarowsky first got interested in language as a Rockefeller Fellow traveling the Himalayas soon after college. Roaming the villages, living among the Nepalis, learning their languages, he grew fascinated by the way different peoples express similar meaning. That passion became his life’s work. He became a computational linguist and uses mathematics, algorithms, and artificial intelligence to map languages to one another. But mapping of words is just “scaffolding,” as Yarowsky puts it. He is more deeply interested in semantics and the meaning of language.
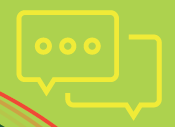
To illustrate, Yarowsky turns to one of the Bible’s shortest but best-known verses, “David killed Goliath.” In his hands, those Hemingway-esque words reveal layers of meaning. Speaking the verse in Japanese, Yarowsky intones, “Dabide ga goriate wo taoshita.”
In a few words, we not only learn that David is “Dabide” in Japanese, but Goliath is “Goriate” and killed is “taoshita” by word-aligning the two languages as in Figure 1. We also learn that in Japanese, the actor (the person who’s doing the killing) is denoted with a “ga.” “Dabide ga” is the killer. The subject of the action, the slain giant, is denoted with a “wo”—“Goriate wo.” And that “taoshita” is a modification of the verb “taosu”—to kill—placing the action in the past tense, Yarowsky says.
Yarowsky is not doing such analyses for Japanese alone, nor for 10, 20, 100, or even 500 languages. “We’re doing this for all 1,600 Bible translations,” he says. “Essentially, every language that has a written form.”
For Yarowsky, each of the Bible’s many translations is a neatly mapped, word-for-word, sentence-for-sentence, verse-for-verse database upon which to train his algorithms. There are even 24 English variations of the Bible from which to draw meaning.
“It’s like 1,600 Rosetta Stones,” he says. “Each with hundreds of thousands of words of English and translated word for word in all those languages. In the linguistic context, it’s actually not a lot of data, but it’s enough to do a lot.”
WELCOME TO THE MACHINE
The joke around CLSP is that faculty member Philipp Koehn wrote the book on machine translation—literally. In fact, he wrote two. The first was his foundational 2009 textbook Statistical Machine Translation and the second was 2020’s Neural Machine Translation.
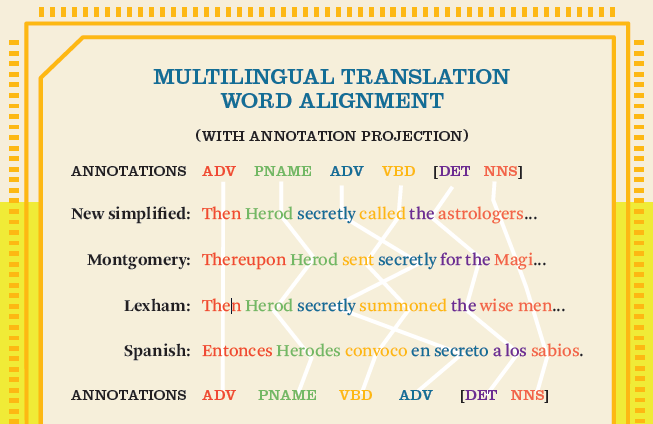
Koehn pioneered a now widely adopted approach known as phrase-based translation that looks to translate small groups of words instead of individual words. He is also developer of the open source Moses statistical machine translation system. Statistical models use word and phrase probabilities to achieve translation. They were the dominant approach in machine translation until only the past few years, when neural network- based models ascended.
“The sea change from statistical to neural models happened almost overnight. Now, neural machine translation is used almost exclusively,” says Koehn, a professor of computer science.
Some of Koehn’s most recent work focuses on a many-to-many translation approach known as multilingual machine translation, which translates among three or more languages at the same time. One of his latest publications explores how lower-resourced languages might benefit. The grouping of similar but lesser-known languages capitalizes on linguistic overlaps and expands resources by sharing data from closely related languages. A machine translation system trained to negotiate between English and two related languages, say Assamese and Bengali, will also improve translation between Assamese and Bengali, Koehn’s research shows.
For Koehn, the challenge is similarly one of finding good data in quantity. He says that it takes as many as 10 million words to build a good model—the more, the better. But the typical novel is just 200,000 words. In that regard, one of Koehn’s go-to resources is recordings and written proceedings of European Parliament in which members speak and submit documents in any of the EU’s 24 native tongues, which are then translated into English, the official language of the EU, and the others. The archives of the European Parliament consist of about 15 million words at present.
“I can imagine a day when we have automatic and instantaneous machine translations between almost any language,” Koehn says.
SHARED VECTORS
The greatest challenge with underresourced languages is that often the word pairs between two languages have not been formally established, says Kelly Marchisio, a doctoral candidate in Koehn’s group who is finding ways to translate unfamiliar languages sight unseen.
It’s a strategy known as “bilingual lexicon induction,” that uses AI and math to establish word pairs. Given a large amount of English text and a similarly large amount in any another language, Marchisio can calculate word pairs in languages she’s never studied before.
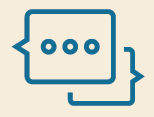
“Imagine I talk to you for 10 hours in English about a random topic,” Marchisio says, laying out a hypothetical. “Then I switch to German, which you don’t speak, and talk for another 10 hours on a completely different topic. With just that amount of data, we can write programs that learn to translate between English and German without any interceding examples of translation.”
In technical terms, Marchisio uses neural networks to calculate a series of mathematical parameters for each word—she calls groups of these parameters “vectors.” Each word in each language is assigned its own vector. She then organizes the terms mathematically in multidimensional digital space, like big balloons of interconnected words where all words of similar meaning in both languages get grouped near one another: colors, animals, travel, weather, and so forth.
“If ‘brown’ and ‘fox’ are often used in conjunction in English, then they are likely to be similarly combined in German, as well,” Marchisio says. With additional analysis, she can start to pair words from both languages.
On the precipice of earning her doctorate, Marchisio says she likes the democratizing nature of her work and helping to keep languages alive. Ideally, she hopes to bring the power of the internet and artificial intelligence to underserved peoples.
“Everybody deserves access to information,” Marchisio says. “And they should be able do it in their native language.”
TRANSLATING AT THE SPEED OF SOUND
Matthew Wiesner’s Haitian relief example highlights the broad complexity of language processing. His realm is the spoken word, which introduces wholly new sets of challenges to CLSP. The frustrations experienced in Haiti birthed the Defense Advanced Research Projects Agency’s LoReLEI, an effort to develop a language processing technology that works quickly, in a matter of hours, even with a language never heard before. Such capabilities hold great promise in low-resource language settings.
“Instead of working on Swahili, Zulu, Telugu, or Bengali, all of a sudden, we were working in Ilocano, spoken in the Philippines. Oromo, the second or third language of Ethiopia. Odia, the eighth or ninth language of India,” Wiesner recalls. “Or, as in Haiti, Creole.”
These emergency approaches don’t need to be perfect, Wiesner says, they just need to work, and work quickly. He uses an approach known as cross-language modeling. In this strategy, AI helps to polish recorded speech, removing the unusable artifacts in the audio—background noise, the emotion of the speaker, and so forth.
The focus is strictly on the phonetics. Given a relatively few bits of recorded content, Wiesner builds a phonetic library of all the sounds in a language (the phonemes, in the linguist’s terminology) and assigns them unique characters (the graphemes). This allows him to transcribe longer recorded pieces phonetically.
“If you give me a recording of 30 minutes of someone speaking a language I’ve never heard before, in less that 24 hours we can create a transcription model that gets half the words right.” — MATTHEW WIESNER
“For instance, in English we have two ‘P’ sounds,” Wiesner explains. “The P sound at the beginning of words, like ‘please’ where there’s a little H after the P,” he says, emphasizing the pronunciation, ‘puh-lease.’ “And then there’s the P sound after an S as in ‘spin.’ We need a way to write both.”
With these phonetic transcripts, Wiesner does not care about spelling or even where one word stops and another starts. He just wants accurate and consistent phonetic spellings. Then, as with printed text, those phonetic spellings serve as patterns to which neural networks can apply traditional language processing techniques.
Wiesner has found that these rapid cross-language strategies work best by pooling all graphemes of all languages in a single universal library—one collection of all the spoken sounds of all the world’s languages.
“If you give me a recording of 30 minutes of someone speaking a language I’ve never heard before, in less that 24 hours, we can create a transcription model that gets half the words right,” Wiesner says. “It’s not perfect, but it doesn’t need to be to work in an emergency situation where understanding is more important that accuracy.”
WORKSHOPPING IT
Despite these collective efforts at CLSP and tremendous progress across the field in general, language processing remains a complex, evolving landscape with many unanswered questions and areas of opportunity. The challenges are so vast, in fact, that Khudanpur has added the role of convener to his already-considerable duties. Since 1995, the CLSP has been bringing together like-minded researchers and professionals from around the language processing world for the Frederick Jelinek Summer Workshop in Speech and Language Technology.
In these on-site, in-person workshops, teams of experts engage in a friendly competition to tackle the thorniest challenges and most promising unexplored avenues in the field.
“We get the best people in the world together for two months every summer to wrestle with some big problems,” Khudanpur says. “Together, we can accomplish in two months three to four years of progress.”
The workshop, named in honor of the former leader of CLSP, Frederick Jelinek, and traditionally located in Baltimore, will be held this summer in France on the campus of Le Mans University.
“It’s dream-team thinking in a work-hard, play-hard environment populated by 40 or so leading experts from academia and industry,” Khudanpur says. “It’s great fun, but also there is a lot of serious work getting done.”
FROM THE MOUTHS OF BABES
One curious phenomenon emerging from such intense study of languages is that all these carefully trained voice recognition and transcription algorithms often fail when the speaker is a child. Paola Garcia-Perera, assistant research scientist in CLSP, came to understand this well when she was doing diarization of children’s speech for use in child psychology research. Diarization involves recording and transcribing children’s speech for hours at a time to search for clues as to how children think, learn, and interact with the world.
Time and again, Garcia-Perera noticed that the resulting transcriptions were not very good; in fact, they were often unusable. Part of the challenge is a technical one, having to do with the difficulty of recording clean audio from a child running about and playing. But there is also an inescapable linguistic element, something in the children’s speech patterns that was tripping up the voice recognition algorithms.
“Children’s speech patterns are different enough to fool the machines,” Garcia-Perera says. “The voice recognition systems that we had were failing, because when a child speaks, they elongate, repeat, and sometimes skip words, or their grammar is still developing.”
Through much trial and error, Garcia-Perera has been able to improve the transcriptions only to be confronted by a challenge of a different sort. Half the world’s population is bilingual. Many bilingual children often engage in “code switching”—moving effortlessly between two languages, mid-sentence.
Accurately transcribing code-switching requires a combined technique called language derivation where the algorithm must determine which language the child is speaking word by word to improve results.
“I think derivation technologies could have broader application in places like Africa, India, and Singapore where the people speak more than one language,” Garcia-Perera says.